
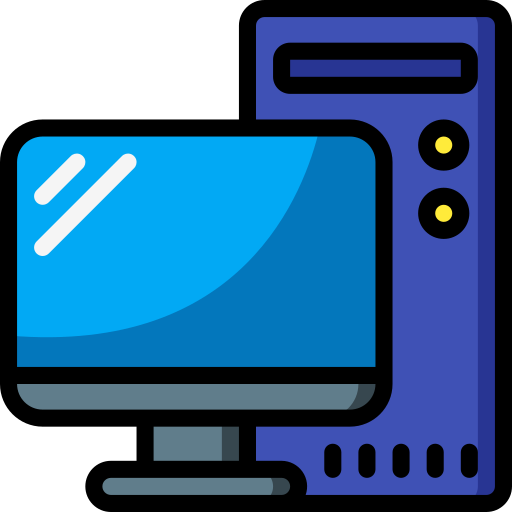
I like draw.io for process diagrams.
I like draw.io for process diagrams.
You mention ThePicardManeuver but leave out Stamets@lemmy.world???
Unused kitty litter works great.
Used kitty litter probably also works. Hey, I don’t judge others’ fetishes.
Xean Connery hates this simple trick.
deleted by creator
Because starting with ‘X’ does not guarantee the ‘sh’ sound. See ‘xylophone’, ‘Xavier’, ‘Xenon’.
Xitter looks like ‘exiter’ to me.
LoRA models still have the underlying fully trained base model underneath; it is not a complete replacement or complete modification of the model weights.
A true Debian user would never tell us that they use Debian. They would say they use Debian Testing’. BTW.
Fungi are pretty awesome. We can decompose plastic with them. Engage in inter dimensional astral travel with them. And have a nice trip by a campfire without ever leaving the chair.
Hooker With a Penis
deleted by creator
His newest social media venture is focused on a niche of extreme exploration, named the Xtreme Network for Xtraordinary Xploration. You can find it at xnxx.com.
Agreed.
It’s not a fair comparison then is it? $80/hr is an expensive but not outrageously so handyman, plus they have their own tools to purchase and maintain and other business operating overhead (fuel and transportation maintenance) etc.
DIY—if you’re able—is always less expensive.
It’s a for-profit service that people use to rent-out, and rent-in their tools. Not a true library so to speak but seeks to accomplish the same. Except that people charging $20/day to rent their battery-powered Ryobi drill is absurd.
There is a “tool library” sort of service (for profit) operating in my area. The prices are absurd—people are charging like $20/day for a tool that would cost $100 new, or half that used on craigslist. My projects often span multiple days, especially if there’s an unforeseen delay—which there always is because I’m a good engineer but a shitty carpenter.
I don’t use the service. I’m all for communal ownership but it still has to make sense.
Hey @Mistral@Lemmings.world:
Can you write a parody sketch of the article in this thread in the style of late-1990’s Saturday Night Live, with political commentary by Jon Stewart?
Have you taught them any tricks?
Some people use git to flog, though. See
git blame
Also PRISM. Maybe the third—wait, wrong side of the array—worst.